A contract and engineering-design manufacturer for medical device OEMs implemented an automated process that incorporates deep learning to inspect fastening hardware on metal carts used to hold kidney dialysis machines.
The company, MPE (Milwaukee, WI, USA; https://mpe-inc.com/), previously inspected the hardware on the carts manually. To do so, operators in the plant inspected carts at the end of the production process. They would walk around all four sides of the cart with a paper checklist and inspect 126 pieces of fastening hardware on the metal carts—a process that took about two minutes to complete. Medical Device Trolley
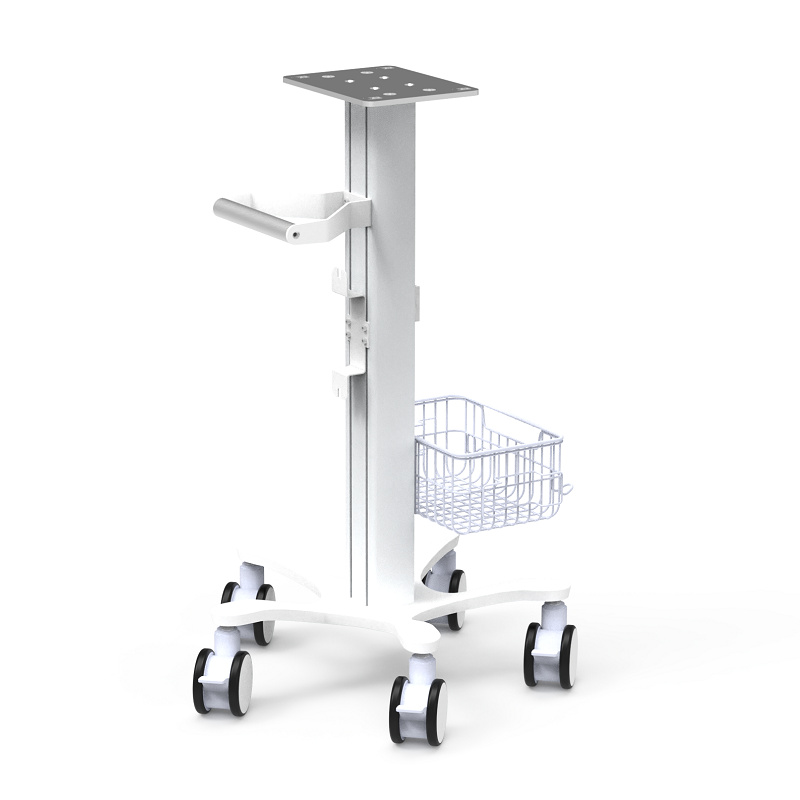
“Once in a while when you’re inspecting different points on a cart that’s being made every few minutes, you’re going to miss some,” explains Austin Appel, co-founder at Overview.ai (San Francisco, CA, USA; https://overview.ai/), which markets the AI inspection system used at MPE.
Erik Kremers, Quality Engineer at MPE, says if inspectors don’t catch a defective or missing part, “that’s obviously very bad for the customer, or if it gets out in the field it could cause some serious issues if there is something wrong structurally with the unit.”
MPE ships the carts to OEMs, and they then assemble the complete unit—i.e., cart and dialysis machine. With the manual process, shipments were sometimes returned to MPE because of a problem with the fastening hardware. Shipping the carts back to the plant, fixing them, and then shipping them back to the customer is a costly proposition, Kremers notes. “It’s not very cost friendly going back and forth,” he says. In addition, he says, the customer often assesses chargebacks to MPE to cover the costs of inspecting an entire lot of carts.
When carts were returned, MPE didn’t have a visual record it could review to determine whether the problem happened before or after the carts left the plant. For example, a cart could be damaged during shipping to the OEM, during assembly at the OEM’s plant, or when the complete product is shipped to an end customer, Appel says.
To solve these problems, managers at MPE in 2021 decided to implement an automated inspection system for the fasteners on the carts. They had two main goals for the automated process: increase the accuracy and speed of inspections and produce electronic image-based documentation, demonstrating that each piece of fastening hardware on a cart passed inspection.
Inspection speed was less of an issue because the overall number of carts produced for kidney dialysis machines each year is relatively low—or about 1,000 carts, Appel says.
MPE purchased an automated solution, the Snap Platform, from Overview.ai. The solution includes a camera, IPC, monitor, and software (image processing and inspection algorithms as well as user interface). The system uses ambient lighting.
The system was installed at the end of the production line, and the cart is rotated automatically to capture four images: one of each side of the cart.
The Snap Platform then runs an algorithm that checks each of the 126 hardware fasteners. The operator will get an overall result, such as 126 passes. If the platform detects a failure, the operator can see exactly where the problem is. That’s because the software displays the images—with each piece of fastening hardware clearly visible—on the monitor.
“The whole thing takes about 20 seconds,” Appel says. “Both the image pre-processing and the AI inference are all done within our software,” Appel says.
Kremers says plant employees still manually inspect components that the camera can’t see, such as if they are hidden within the assembly, but the software “significantly reduces the number of things we have to check.”
Appel adds, “I think one of the things that's interesting and worth highlighting is that typically metal components are pretty hard to inspect. Obviously, they're very reflective. A piece of fastening hardware on an a-frame like that would be pretty tough for a traditional vision system.”
With traditional machine vision, Appel says the integrator would need to build a system for each fastener, and each one would need a light source and a camera. “Your hardware cost would be crazy. Your development cost would be crazy. It wouldn’t really be an economical system.”
Appel says development of an inspection system based on deep learning models tends to be faster, too, because a developer does not need to hard code a lot of rules to judge whether a piece of fastening hardware passes or fails the inspection.
The system from Overview.ai includes a deep learning algorithm that MPE needed to customize for its product. which would typically require quite a few images to show the algorithm what a good piece of fastening hardware looks like.
The problem was the low volume—about one per month—of defective pieces of hardware. “That’s not enough,” Appel says.
To solve the problem, Overview.ai used a method called segmentation because it requires fewer than 10 images per region of interest. With segmentation, “you highlight certain pixels within an image as interesting,” Appel says. For example, you might highlight 100 pixels within a larger image and label it as a scratch.
For its part, MPE created defects on purpose, so that it could feed the algorithm the necessary training images.
Since installing the software to inspect the carts used for dialysis machines, MPE created a similar automated inspection process using the Snap Platform for two other types of carts. One of the carts is used to house a robotic arm used during surgery and a second cart holds the monitor and other communications equipment that surgeons use during surgery to control the robot.
For all three carts, the automated system has dramatically improved the inspection process and the number of problematic units that reach MPE’s customers.
In situations in which a string of complaints about the same issue occurs, MPE usually revises the software to address the problem. For example, “we recently implemented an update to our surgical cart line inspection. We had a string of complaints regarding three different components being missing. The update added a check for these three components and these complaints have been eliminated since,” Kremers says.
Overall, Kremers sums up the value of the automated inspection system, saying “It saves you time and improves the quality of the product. I hear fewer complaints from the customer.”
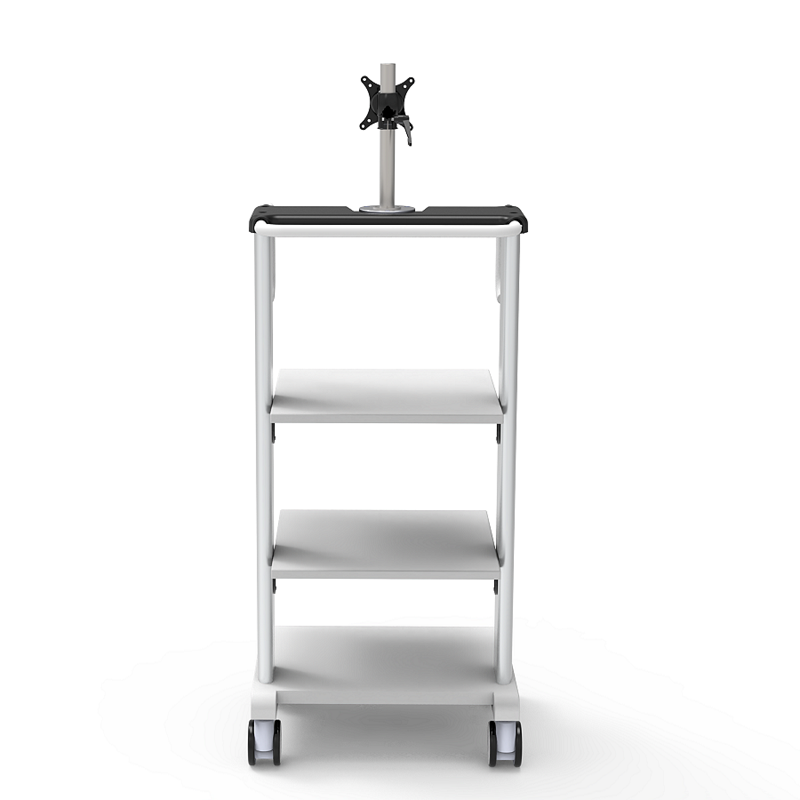
Corner Wall Mount Linda Wilson joined the team at Vision Systems Design in 2022. She has more than 25 years of experience in B2B publishing and has written for numerous publications, including Modern Healthcare, InformationWeek, Computerworld, Health Data Management, and many others. Before joining VSD, she was the senior editor at Medical Laboratory Observer, a sister publication to VSD.